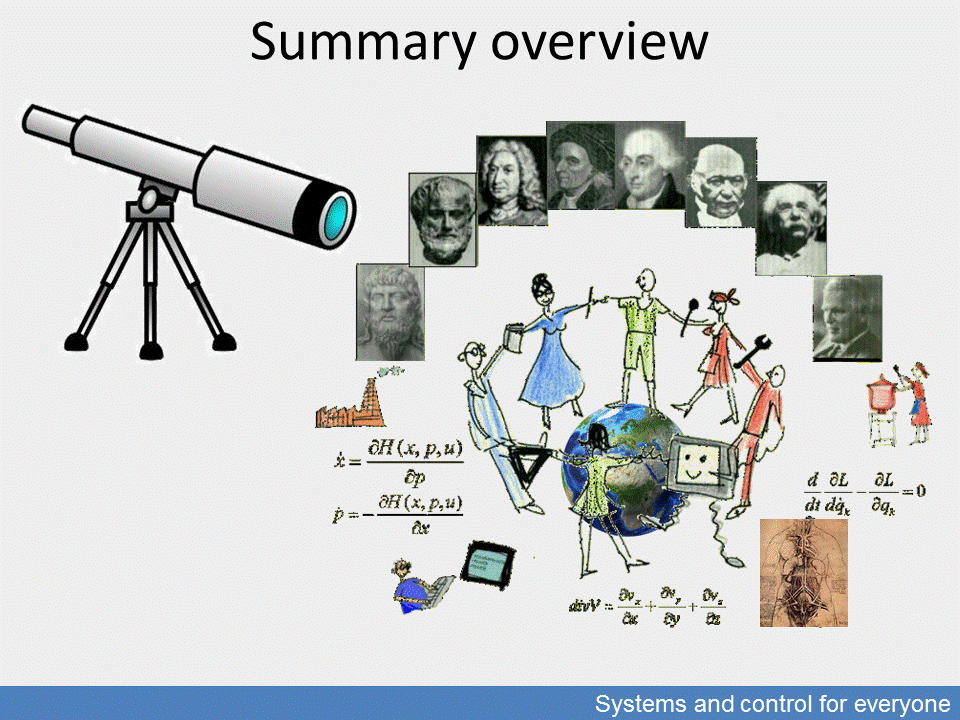
When dealing with this unifying, development-oriented approach, it is worth having a look at the history of controls, which necessarily implies the repetition of what we have already discussed, but in a new context.
The basic goal of controlling systems is to ensure their stability. This should be borne in mind when trying to achieve the quality characteristics that reach the goals of the system, and when trying to ensure that the system will not become unstable as a result of expected internal and external disturbances and any deviation from the default settings, and will not substantially differ from the desirable quality characteristics.
Mathematical-computational models were created to be able to examine system behaviour and design systems. As a basic problem, system dynamics is usually non-linear, while our computational tools are based on linear assumptions. This restriction becomes particularly significant when modelling highly complex systems as the superposition resulting from the linear condition is not valid to non-linear relations.
Although the concepts of complex numbers were already appeared in the 16th century and the concepts of complex analysis in the 18th century, Newtonian dynamics disregarded them, and even the first theoretical models created for regulated steam engines in the 19th century went without them. It was the transmission technology of the 20th century and the control tasks of World War II that first called for the application of functions of a complex variable. The first major achievements were induced by a complex problem of the two task groups, namely the surveillance radar guidance. These achievements form the foundations of the techniques used in today’s control engineering practice.
The complex variable representation led to the dual (time- and frequency) representation of system dynamics, to the rich analysis and synthesis of the identities and characteristics that allow for different usage, and thus to the massive extension of the original Newtonian representation.
The residuum theorem in complex analysis as the mathematical representation of the Stokes-Gauss fluid mechanics became the theoretical and calculation basis of the stability relations in classical control theory. This relates to the interpretations of dynamic processes described by analytical functions, and the role of poles, the roots of the characteristic equations of linear differential equations in the examination of stability.
Approximation procedures evolved as systems and the related tasks became more and more complex.
As the complexity of tasks increased, it became clear that the input-output relations of traditional methods do not always provide appropriate information about the state of systems. This realisation led to the conceptual system of state spaces, to the canonical equations and of handling input-output and the measureable or estimated state variables in a uniform way.
State equations represented in matrix forms became the basic methods of further calculations, handling of linear systems and approximation of nonlinearities.
State descriptions suggested the conceptual and calculation conditions of controllability and observability. Observability examines whether the original values of state variables can be determined from the measured output data, while controllability examines whether it is possible to intervene in the process with the available tools.
State methods included disturbances affecting the systems and suggested filtering methods for them, primarily the Kalman filters.
The process of state-based filtering and its connection to the feedback process made it possible to continuously correct state estimates and monitor relations moderately deviating from the linear.
Quality requirements going beyond stability were yet another milestone in the same classical development process between the 1960s and today. These requirements are described by the usually quadratic norms calculated from the time and frequency processes of the states, and are connected to the effect chain of the control according to the linear conditions. This context is the starting point for determining the stability-preserving parameters of the quality requirement elements, calculated in a way that the elements of the extended system should be relative primes so that they do not affect each other’s stability (Youla parametrisation).
The 1980s presented new challenges: the complexity of systems to be controlled and quality requirements drastically increased, while it was a demand that systems should operate properly even if exposed to expected disturbances and changes in the operation. Another indicator of the changes was the revolutionary growth of the magnitudes of calculation possibilities. All this induced a new way of thinking which was both compatible with and radically different from the previous mindset.
This guiding principle here was to find spaces and space transformations that support the modelling and calculation of these tasks. Abstract spaces were already part of the developments of systems theory, as we had the fundamental experience of two- and three-dimensional spaces and its geometry resulted from our experience of motion. In control theory, initially the metric versions of the Euclidean space, the complex plane, and later the Banach-Hilbert spaces were used.
The main purpose of this introduction was also to illustrate this developing experience of space. The next logical step was the use of projective geometry which, according to the Klein geometry interpretation of the group theory, ensures transformation possibilities giving freedom to calculation methods through representation and calculation similarities. Ancient shadow-casting and star-tracking calculations were precursors in this respect.
Projective geometry defines proportions and motion formulas which provide representation and calculation freedom within the given group of shapes. These change formulas suggest that in complex system representation works the basic image of the feedback control system closed-loop system can be represented with a single simple element and handle this as a term of matrix algebra in mathematical representation.
The very same approach helps the separation of complex system versions to simpler elements, the operations of linear fractional transformations which can be represented using the schemes of state space. Thus, to simplify further steps, the scheme is split into a process loop and a disturbance loop, the K schemes used in the considerations.
For our control tasks, the Möbius transformation and metric Hardy spaces are the most relevant here.
Two metrics are of particular importance from our perspective: the H2 and the Hinf integrals which sum up the quality characteristics and which are valid to transfer functions described both in the time and frequency domains.
The Möbius transformation is the mechanism of shifting, shrinking, growing and rotating which preserves the basic characteristics of linear relations. This is used to transform the half plane indicating stable states of complex variable space running to infinity into the unit circle, that is, into a closed metric which can be comfortably handled in terms of stability and quality control. The properties of the unit circle, or in multi-dimensional cases the unit spheres of Hardy spaces, open up many possibilities which can rely on the former achievements of mathematical analysis.
The analysis of the connected behaviour of complex systems uses the group characteristics and harmonic relations of projective geometry. Deviation from linearity can be handled within the relatively narrower domains of system changes. These domains are divided into parts by measurable and estimable states, supported by the state space approach.
The outlined fractional scheme-formalism and linear approximation enables the handling of matrix algebra and machine simulation programmes, though as complexity increases and the number of conditions grow these also call for trained and experienced professionals.
These methods help the frequently mentioned task of robust control, namely to tolerate deviations and modelling uncertainties and keep the stability and quality operations of the system within definable limits. This is enabled by the linearity conditions and the related small gain theorem, which also suggests that there is still very much theoretical research to perform mainly in the field of design.
Promising research directions include the examination of non-Euclidean, hyperbolic geometries which is a logical continuation of the above discussed approaches, particularly projective geometry. Euclidean parallel trajectories, projection lines converge in these geometries, so they can provide new, more flexible metrics or even more adaptive surfaces for conditions deviating from the linear.