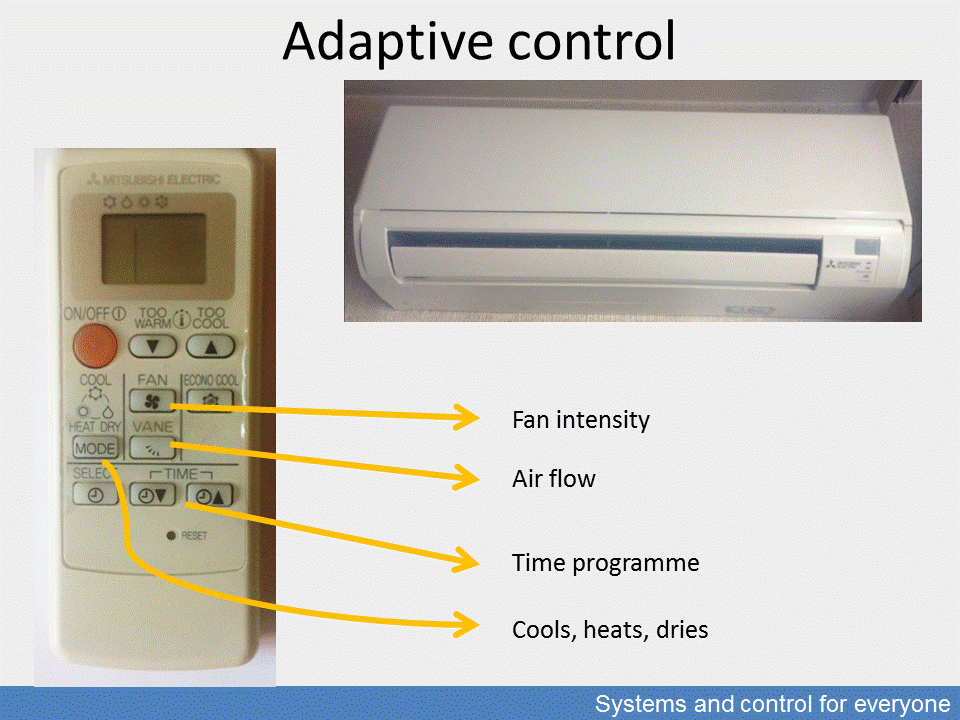
The adaptive controller senses the changes in the state of a system and accordingly adjusts controlled relations to an optimum level. An air conditioner, for example, adjusts its parts responsible for influencing operation in accordance with indoor temperature and humidity.
Adaptive control aims to facilitate adaptation to system changes so that the control remains in line with the requirements in spite of the changes of system parameters.
The system is identified to detect system changes. Mostly, we assume a given structure (e.g. number of time lags, dead time) and determine system parameters in a way that the measure of the difference between the system and the model (e.g. the sum of the squares of differences measured at the sampling points) should reach a minimum value. The controller’s parameters are then adapted to the changed identified model to ensure that control behaviour remains stable and practically unchanged despite the parameter changes.
The implementation of adaptive control raises several problems. Identification means learning the parameters of the process. If the control is operated during the learning process, it can cause oscillation in the control loop. So it is advisable to wait for the system to “learn” the new parameters before using the control. Identification is more difficult in closed loop than in open loop. Identification requires sufficient excitation but in the control loop we try to reach a stable output signal. This state, however, usually does not provide appropriate excitation for the identification. For this reason, it is useful to build a so-called expert system for the adaptive control, which for example performs preliminary identification in an open loop, and then it sets up the control parameters and starts the control process. In this case identification is carried out time to time. Identification and control is operated together carefully.